Hi!
I work on Systems at Substack. You can see some of the projects I’ve worked on in my resume. I am super excited about all things infrastructure engineering, machine learning and running things at scale.
I’ve been fortunate to live in 5 different countries and visit over 50, from early startup work in London and Berlin to settling in San Francisco.
In my free time I enjoy meditation, jhanas, CrossFit and making abstract art. I care deeply that what I do positively affects those around me.
I’m usually at the Thursday sits at the Berkeley Alembic. If our paths cross in meaningful ways, don’t hesitate to reach out!
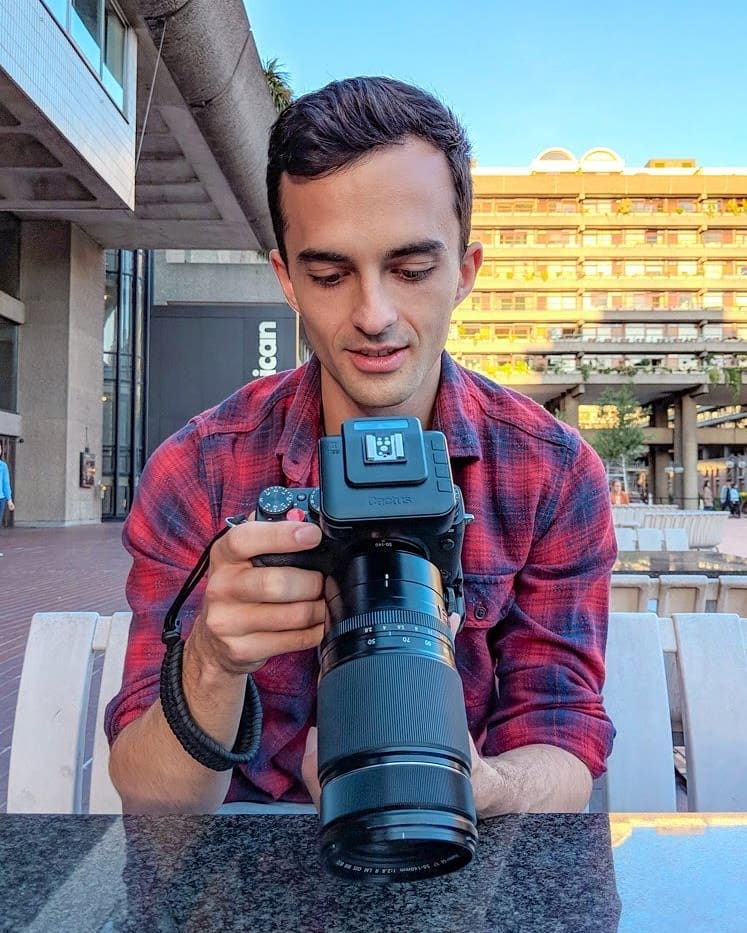
Writing
12 Nov 2023
A collection of engineering principles.
12 Nov 2023
A collection of personal principles.